Large language models (LLMs) are no longer a novelty, but enterprises are still grappling with their effective integration into business applications. While they continue to struggle with implementation challenges such as ROI justification, data security and privacy, and integration within existing infrastructure, they now face the advent of another transformative technology— multimodal AI.
Multimodal AI excels at integrating and processing data from diverse sources, including text, audio, image, and video. This capability allows it to provide more contextual and comprehensive outputs, making it ideal for complex applications such as autonomous vehicles, speech recognition, and emotion recognition.
Multimodal AI is heralded by technology giants betting on it as the future of AI. In March 2023, Baidu’s ERNIE Bot led the charge in commercializing multimodal capabilities for text-to-image and video generation, setting a new benchmark that others strive to meet.
-
- In May 2024, OpenAI launched GPT-4o, a multimodal LLM that uses text, image, audio, and video as input and output.
- In March 2024, HyperGAI launched its multimodal LLM, which accepts text, image, and video inputs. It plans to enable multimodal output generation in the future.
- In December 2023, Google launched the Gemini Pro, a multimodal LLM that supports text, code, audio, image, and video generation.
Why Do Enterprises Need Multimodal AI?
Multimodal AI identifies data relationships across diverse formats, improving semantic coherence among complex data modalities such as videos and audio. The examples below demonstrate how this technology enhances data analysis across industries, providing detailed contextual insights that strengthen decision-making processes.
-
- Complex problem solving: A manufacturer developing autonomous vehicles can use multimodal AI to integrate data from cameras, LiDAR, GPS, and audio sensors to navigate complex urban environments more safely, considering visual cues, spatial information, and even auditory signals such as emergency vehicle sirens.
- Enhanced predictive analytics: Weather forecasting agencies, for instance, can combine satellite images, atmospheric sensor readings, historical weather reports, and video data from weather stations to deliver highly accurate and reliable forecasts. This enables to proactively develop response strategies for weather-related events.
- Hyper-personalized product recommendations: By analyzing customer sentiments from product reviews, styling preferences from product images, and real-time fashion trends from social media, AI can provide highly personalized recommendations that align with each customer’s unique style preferences and current market trends in the fashion industry.
- Enhanced user experience: Multimodal interfaces that combine voice, touch, and gesture recognition create a more natural and intuitive user experience. For instance, a bank can implement a multimodal virtual assistant that accepts voice commands, text input, and gestures, making it easier for customers to access services through their preferred interaction method.
Navigating the Early Challenges
While multimodal models hold immense promise, they are still in their infancy, with ongoing research and experimentation aimed at overcoming significant challenges, as listed below:
-
- Alignment issues: Ensuring that models understand prompt instructions and generate accurate, unbiased outputs requires identifying relationships between multiple modalities. This is complicated by the scarcity of annotated datasets, noisy inputs, and difficulties in comprehending inverted or distorted text, requiring sophisticated preprocessing and alignment techniques to ensure accurate outputs. For instance, aligning scanned images of handwritten notes with typed legal texts poses significant challenges in legal document analysis. Furthermore, noisy inputs such as smudges, varying handwriting styles, and poor scan quality can distort the text, making it difficult for the AI to accurately interpret and align the information.
- Co-learning difficulties: Transferring knowledge across modalities is a major hurdle. For example, sound is less studied than images or text. Integrating haptic data with visual information for robotic manipulation tasks is another instance. To improve learning in this area, researchers are exploring the TactileGCN model to combine tactile sensing data with visual inputs, enhancing the robot’s ability to understand and manipulate objects with a sense of touch.
- Fusion challenges: Combining information from different modalities for prediction is complex due to varying predictive power and noise levels. For instance, in autonomous driving, data from cameras (visual), LiDAR (3D spatial data), and microphones (audio) must be integrated to create a comprehensive understanding of the vehicle’s environment. Effectively merging these modalities involves sophisticated techniques where initial predictions from each modality are combined and refined through multiple processing stages, ensuring better situational awareness and decision-making in dynamic driving conditions.
- Security concerns: Multimodal models are susceptible to adversarial attacks. Images, as inputs, can be exploited in prompt injection attacks, potentially instructing the model to produce harmful content or hate speech. For instance, a malicious actor could introduce an image with subtly embedded adversarial noise in a healthcare AI system that uses both medical images and patient records to diagnose diseases. This tampered image could cause the AI to misdiagnose a condition, potentially leading to incorrect treatment recommendations.
- Curated data requirements: Sourcing high-quality text-image pairs is more challenging than obtaining purely textual data. Detailed descriptions are necessary for meaningful text-image pairs. This requires human annotators, which is costly and time consuming. For example, creating a dataset for autonomous vehicle training involves annotating complex urban scenes with detailed descriptions of various objects, actions, and interactions, demanding extensive manual effort and expertise.
Despite these early challenges, the rapid advancement in multimodal AI continues, driven by the potential to revolutionize numerous applications. As these issues are addressed, the transformative power of multimodal AI will become increasingly accessible and impactful.
The Transformative Potential of Multimodal AI
In the past, enterprises often relied on specialized platforms to address distinct aspects of their business processes. Take, for instance, a global logistics company managing the end-to-end shipment tracking and delivery process, as shown in the figure below.
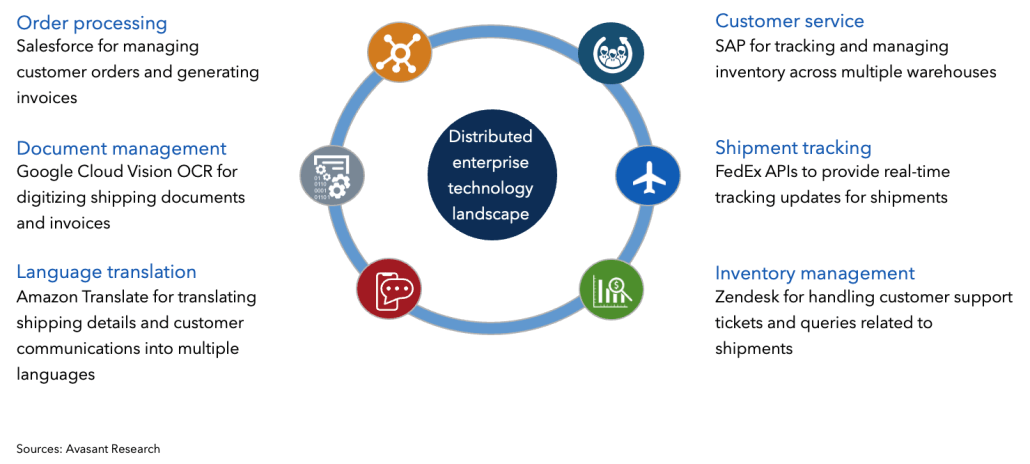
Figure 1: A representation of the enterprise technology landscape without multimodal AI
While each platform was the best in its class, integrating these disparate specialized tools required significant effort. The logistics company needed a robust IT team to manage integrations, ensure data consistency across platforms, and maintain custom APIs to connect these systems. This approach, while effective, led to substantial technical debt, higher operational costs, and complex tool governance programs. The need for continuous monitoring and troubleshooting across multiple platforms further complicated the workflow, necessitating large teams to sustain seamless operations.
But now, a single multimodal AI platform can transform the business workflow of this global logistics company by processing various data modalities through a unified system, significantly reducing complexity and operational costs. From order processing to customer service, multimodal AI seamlessly integrates diverse functionality, such as data extraction from shipping forms and invoices, processing of multilingual shipping information, customer communications, and compliance documents, eliminating the need for separate data management or translation software. This integration extends to inventory management and shipment tracking, where the AI uses real-time data from sensors and shipment logs to provide timely updates on inventory levels and delivery status. Furthermore, multimodal AI can efficiently handle text- and voice-based queries in customer service operations, employing natural language processing to promptly understand and respond to customer needs. This unified approach enhances operational efficiency and improves decision-making by providing comprehensive insights across all operational facets.
Given the transformational impact of multimodal AI, enterprises across sectors are now exploring it, offering a massive opportunity to enhance productivity and gain a competitive edge.
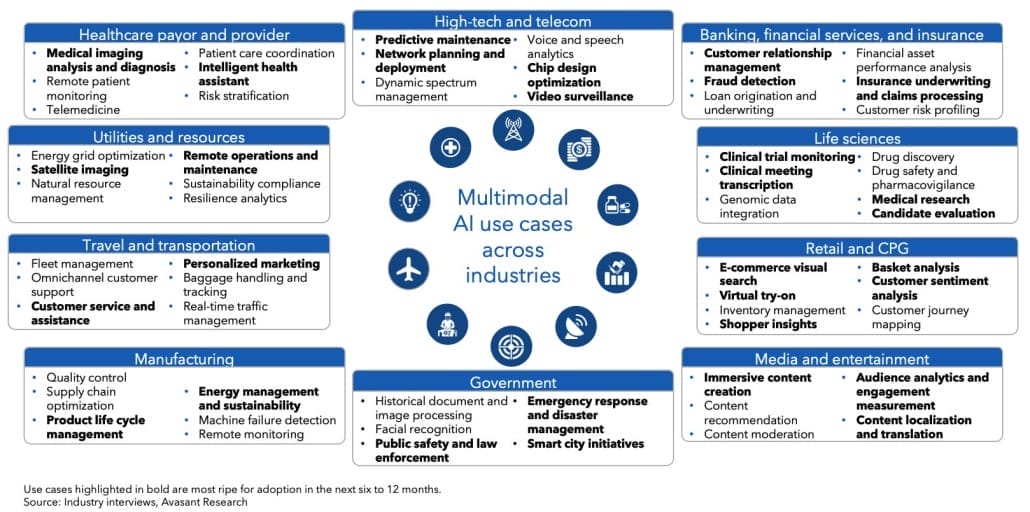
Figure 2: Industry-specific multimodal AI use case representation
Mentioned below are some real-life enterprise use cases that have successfully leveraged multimodal AI capabilities, demonstrating its diverse applications across industries to gain a competitive edge.

Figure 3: Illustrative enterprise examples
Recommendations for Enterprises
While multimodal AI presents numerous opportunities for seamless automation, enabling the vision of straight-through processing that originated with the RPA era, several key considerations must be addressed before embracing multimodality.
-
- ROI evaluation: Before integrating multimodal AI into existing workflows, conducting a comprehensive ROI evaluation is crucial, particularly when replacing cost-effective solutions such as computer vision in non-dynamic use cases such as personal protective equipment kit monitoring. For instance, in manufacturing, computer vision effectively monitors employee adherence to safety protocols. However, multimodal AI could enhance safety monitoring by integrating text-based manuals with video demonstrations in training materials, thereby improving employee engagement and compliance.
- Data privacy and protection: Given the sensitivity of personal data processed by multimodal AI systems, robust measures for data ownership, consent, and protection are essential. For instance, an e-commerce platform must implement stringent privacy policies to safeguard customer information across voice, image, and text interactions, ensuring compliance with data protection regulations such as GDPR.
- Identify complex use cases for experimentation: Multimodal AI significantly broadens automation possibilities beyond traditional AI and RPA capabilities. Enterprises should assess the feasibility of automating complex business workflows that were historically challenging, such as patient care coordination, which involves integrating diverse data modalities such as patient electronic health records, medical imaging scans, textual medical notes, and real-time physiological data from wearable devices.
- Accuracy enhancements: Collaborate with platform vendors with curated datasets tailored to your domain or industry use case to minimize model hallucinations. For example, Microsoft Research leveraged millions of PubMed Central papers to curate 46 million image-text pairs, creating the largest curated biomedical multimodal dataset. The dataset is ideal for disease diagnosis and treatment planning.
- Human in the loop: Enterprises must establish guardrails to prevent high-stakes decisions, such as financial investment strategies, from being entirely automated by multimodal AI. While AI can analyze market trends and examine financial documents to suggest investment opportunities, final decisions on portfolio management should involve human expertise to mitigate financial risks and optimize returns.
By Chandrika Dutt, Associate Research Director, Avasant, and Abhisekh Satapathy, Lead Analyst, Avasant