Generative artificial intelligence (Gen AI) presents exciting opportunities for enterprise IT. Machines can produce extraordinarily creative and realistic content, from marketing copy to original music. Gen AI has the potential to be a powerful tool, amplifying human creativity and boosting productivity. However, Gen AI has its own set of difficulties that must be carefully considered.
Misinformation can be disseminated and deepfakes can be produced by malicious actors using Gen AI, while biases in training datasets can lead to discriminatory results. Additionally, when AI produces content that is similar to previously published works, copyright infringement becomes a worry. Even when Gen AI is used responsibly and accurately, like all technology, as it proliferates, it will be important to track costs, manage the number and type of AI in use, and provide solid policies to make the most of the technology. To ensure responsible development and use, strong governance frameworks are crucial. Enterprises must employ strategies like dataset curation practices, bias detection algorithms, and clear attribution guidelines to help mitigate these risks.
One of the most concerning aspects of bias in Gen AI is that it can be subtle and represent latent prejudices in the training set. Prioritizing diversity in datasets is necessary to reduce this. For text generation, this means incorporating a broad range of writing styles and viewpoints. Imagine training AI with only historical texts; the results could reinforce antiquated prejudices. Similarly, to prevent AI from acquiring the stylistic peculiarities (and possible prejudices) of a single programmer, enterprises should source code from multiple developers while creating AI systems. For image synthesis, we must ensure that AI learns to appropriately portray individuals and scenes, regardless of their ethnicity, gender, or background.
Human oversight is equally important. Curators with a keen eye can identify and remove biased content before it influences AI. For example, exposing an AI program to news articles that propagate stereotypes about a certain demographic will train it to do the same. To further mitigate bias, tracking the origin of training data allows for further checks, ensuring the source material itself is not riddled with hidden biases. Ideally, AI should be capable of producing news pieces with a global viewpoint, biased-free code, or images that highlight diversity.
There are two major areas of AI governance to consider. First, there is the ethical and accurate use of Gen AI. The second is more focused on enterprise strategy and effectiveness.
Ethical Use of AI and Data Curation
At the beginning of any Gen AI project is the selection of AI, the data it can access and train on, and the methods put in place to be sure that the AI is accurately reflecting its training. The data curation and quality control aspects are crucial to gaining the most business value out of the Gen AI initiative. AI, like other technologies, is a “garbage in, garbage out” proposition. Despite the name, AI seldom invents new ideas. It pulls together ideas from across its source data and synthesizes them. Data curation can help keep AI “on task” and better reflect the IP and data you have already collected.
While data curation is essential, some biases might sneak through. This is where bias detection algorithms can be powerful tools for further refinement. These algorithms excel at two key functions. First, they can identify statistical patterns that indicate bias in text generation, code creation, or image synthesis. For instance, an algorithm might flag code that shows racial biases in decision-making logic, text that regularly uses gendered language for certain professions, or images containing stereotypical portrayals of faces and scenes.
Second, through feedback loops where human-identified biases are fed back into the system, these algorithms can learn to identify and highlight similar patterns in the future. This continuous learning process progressively improves the AI outputs, making such programs fairer and less vulnerable to biases that might linger in the training set.
Data curation and bias detection algorithms are crucial tools, but attributing AI-generated content can be an effective technique for spotting and reducing bias in the long term. While attributing AI-generated content would not eliminate bias in training data, the labeling of content as AI-generated or the disclosure of the training data sources used should be mandatory. This transparency can empower users to become critical consumers of information. Standardized watermarking, text labeling, or file formats for AI-created content can achieve this.This informs users that they are interacting with AI-generated text, code, or images. Users are further empowered to become critical consumers by challenging any potential biases in the content using standard disclosure language.
Clear attribution goes beyond just informing users. It also makes audits and bias detection easier. Defined attribution rules, which may include giving credit to the AI tool or source, allow developers to track any biases back to the training data source by using. Consider it a fingerprint that can be tracked. By keeping track of the training data used for certain AI models, developers can identify and correct sources of bias in their datasets during audits.
Although clear attribution is a useful technique, it is important to recognize its limitations. Users may choose to just ignore disclosure labels because they are confused or overwhelmed with information. It can also be difficult to attribute complicated AI models with several contributing components. For example, it would not be correct to attribute an AI art generator that finds inspiration from just one source if it took cues from a variety of historical artistic styles.
While data curation and clear attribution are crucial for responsible AI development, enterprises also need strong governance frameworks to dictate what kind of data large language models (LLMs) can be trained on.
-
- Data source vetting: Frameworks can set standards for screening data sources before they are used to train LLMs. This can entail evaluating the information for any biases, copyright violations, and security issues. Consider using an LLM to summarize news stories; data sources would be carefully examined for possible political bias and factual correctness.
- Data labeling and anonymization: Frameworks could require training data to be labeled to classify its origin and content. Sensitive data may also need to be anonymized to preserve privacy. An LLM for medical diagnosis, for example, might require that patient data used for training be made anonymous.
- Human oversight and review boards: Expert-led independent review boards could be formed to supervise the data selection procedure and provide guidance on data governance procedures. These boards can guarantee that the development of ethical AI and responsible data use are in sync.
Enterprise Governance for Deployment of AI
Enterprise IT departments can benefit from public and private AI tools, but careful standards are needed to guarantee their ethical and successful integration. In addition, like the adoption of any other technology, it needs to be monitored to meet budget needs, achieve a solid ROI, and be adequately integrated into business practices.
-
- Understanding use cases and capabilities: The capabilities and constraints of available AI tools vary. To ascertain whether an AI tool is in line with the requirements of the organization, a thorough assessment is required. For example, an IT department entrusted with automating the development of social media material would have to evaluate the text generation capabilities of an AI tool to make sure they align with the language and style of the brand. As use cases abound, it might be tempting to “throw AI” at every problem. Selecting the places it can be applied to make the most ROI is crucial.
- Data security and privacy: When it comes to data security and privacy, public AI tools could differ from proprietary ones. Before incorporating any AI tools into internal business processes, IT departments should thoroughly examine the data handling policies of these platforms. This could entail ensuring data privacy laws are followed and, if needed, extra security measures should be implemented.
- Alignment with governance frameworks: The data curation, bias detection, and attribution practices discussed earlier apply equally to the use of public AI tools. Enterprise IT departments ought to make certain that their utilization of open-source AI technologies aligns with the organization’s broader AI governance policies. When it comes to following attribution requirements, public AI tools can be especially helpful because of their open nature, which frequently makes it easy to identify the underlying algorithms and training data.
- Futureproof your AI governance: Most companies are just beginning their AI journey. However, as AI grows in scope, enterprises should be prepared for the day human intervention or partnership with AI is no longer required. AI or “agents” will begin talking to each other without the need for humans in at least some steps. Governance frameworks should be built to withstand the growth of AI/agents so that secure networks of AI bots and agents can work together, perhaps across multiple stakeholders including suppliers, partners, and even customers.
The Strategic Value of Governance
As we have discussed, strong governance frameworks are essential for assuring the ethical development and application of Gen AI as well as effective business use. Gen AI can be deployed within any department or function of an organization. As a cross-functional, potentially paradigm-shifting technology, it is important to understand who is overseeing the Gen AI mission. Is governance centralized with one organizational unit, is it decentralized across multiple units, or is there some combination?
As shown in Figure 1, 41% of companies say Gen AI governance is centralized in their organization. Another 35% say they have a federated model, which can be defined as a central group that approves business unit projects, but each business unit is responsible for its own implementation. Another 20% say they have a decentralized model where each department or line of business makes its own decisions about Gen AI and carries them out. Another 4% have no formal policy in place.
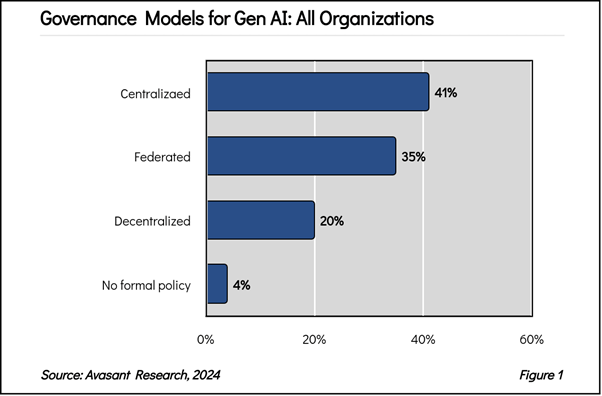
Figure 1: Governance Models for Gen AI: All Organizations
The governance structure that an organization chooses has a big influence on how it uses Gen AI. A centralized approach offers consistency and aligns with the company’s goals. However, within individual departments, it can still lead to bottlenecks and hinder innovation. On the flip side, while a decentralized model fosters agility and experimentation, without adequate controls, a decentralized model may lead to ethical issues and inconsistencies. The federated model, though, attempts to strike a balance, offering central oversight while empowering business units.
Ultimately, an organization’s size, culture, and risk tolerance will determine the best governance structure. Whatever the model that is used, ensuring the responsible and ethical development and application of Gen AI requires well-defined policies, transparent lines of accountability, and constant communication.
Although Gen AI has enormous potential, responsible development and application are crucial. By integrating data curation techniques, rigorous governance structures, explicit attribution criteria, and bias detection algorithms, we can ensure Gen AI is a tool for advancement rather than discrimination. Public AI can greatly aid the democratization of AI, but appropriate use policies and user knowledge are necessary. To fully realize the potential of this strong technology, we must continue to promote openness, cooperation, and dedication to the development of ethical AI.
By Asif Cassim, Principal Analyst, Avasant