Over the past three decades, we have witnessed a remarkable evolution from back-office to middle-office and now front-office automation. This journey, driven by advancements in AI, has brought us to the cusp of a new era in business automation.
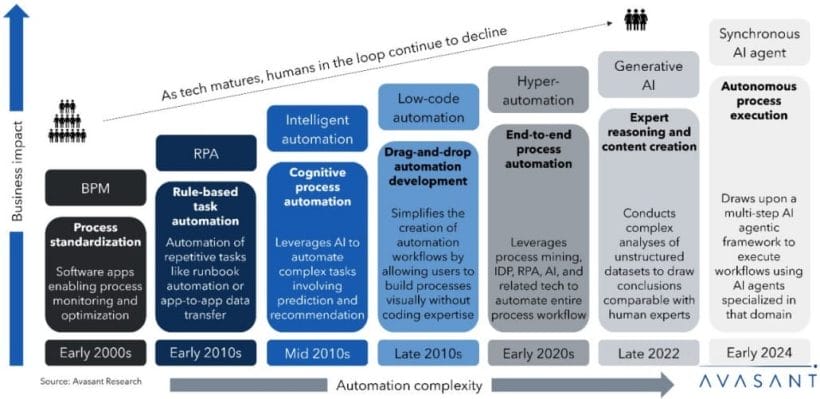
Figure 1: Industry shifts to synchronous AI agents, reducing human involvement in complex workflows
Initially, the focus was on business process management (BPM), with vendors like IBM and Appian developing tools to monitor, re-engineer, and optimize processes. However, the automation landscape began to shift with the advent of robotic process automation (RPA), leading to the rise of specialized players such as Automation Anywhere and UiPath.
RPA was a game changer, offering attended automation solutions that could handle routine, rule-based tasks efficiently. But automation did not stop there. The ecosystem began to expand rapidly as complementary technologies such as process mining, conversational AI, intelligent document processing, and workflow orchestration emerged. Together, they laid the foundation for what is now known as hyperautomation—the orchestration of multiple automation technologies working in concert to execute entire processes end to end, with minimal human intervention.
The democratization of automation saw its next major shift with the introduction of low-code automation platforms from vendors like Nintex and Appian, which enabled non-technical users to design their automation workflows. While this opened new possibilities, low-code platforms were largely confined to solving simple tasks rather than executing complex, multilayered processes. Automation of complex workflows, especially those requiring deep AI capabilities, remained challenging, often involving hard-coded solutions despite advances in AI such as natural language processing and neural networks. While AI proved invaluable in predictive processes like fraud detection and loan approval, fully autonomous automation of complex workflows remained elusive.
We are now entering the era of synchronous AI agents, where the vision is to use specialized AI agents to handle end-to-end execution of complex processes, reducing human oversight to just approvals and governance. However, this vision remains aspirational for now. While synchronous AI agents promise to exclude humans from much of the execution path, their practical implementation still faces hurdles, such as ensuring reliability and overcoming the complexities of contextual understanding.
The automation fabrics of the future will likely combine RPA, AI copilots, and synchronous AI agents, coexisting and complementing each other. One such scenario is customer order returns management. Let’s look at how these technologies work collaboratively, leveraging their unique strengths to fully automate order returns while minimizing human errors, manual work, and time to completion.
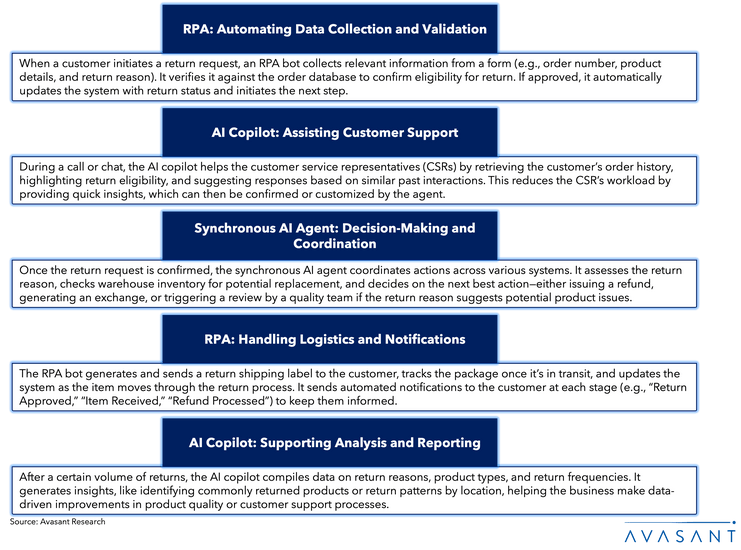
Figure 2: Illustration of RPA, AI copilots, and synchronous AI agents working together in the order return management process
Market Dynamics
Whenever a new technology emerges, businesses pivot their business models to reflect the latest capabilities in their offerings, whether through acquisitions or by forging strong partnerships, to stay competitive.
The business process automation space is no exception, having undergone significant consolidation over the past few years. Many specialist startups in areas like RPA, conversational AI, process mining, and intelligent document processing (IDP) initially struggled to scale standalone product sales. This has led larger players to step in, aiming to offer end-to-end automation solutions or integrate these emerging technologies into broader enterprise systems like ERP, CRM, and content management platforms.
For instance, in 2020, Hyland acquired Another Monday to strengthen its automation capabilities and complement its content services portfolio. Similarly, Microsoft’s acquisition of Softomotive that same year expanded its low-code RPA capabilities within Power Automate, a cloud-based platform that allows users to create automated workflows across applications. This consolidation is a recurring pattern in tech, and synchronous AI agents seem set to follow a similar trajectory.
Salesforce, a cornerstone of enterprise software, recently announced a bold pivot toward AI agents, essentially positioning itself as a startup again in this space after 25 years of focusing on CRM. Other companies are making similar moves: SoundHound, known for its voice AI, acquired Amelia in August 2024 to break into the AI agent business, while Sema4.ai bought the automation vendor Robocorp earlier this year to build AI agents. With AI agents becoming the next frontier, both AI-native firms and traditional software companies are venturing into this space, determined to capitalize on the growing demand for automation that extends beyond traditional processes.
Even established automation giants like UiPath and Automation Anywhere are feeling the pressure to innovate. Following Blue Prism’s acquisition by SS&C, there has been notable customer churn, with many switching to Microsoft Power Automate for its cost advantages. However, for more complex workflows, UiPath and Automation Anywhere continue to dominate, but their high platform licensing fees and implementation costs continue to be an enterprise concern. In a typical RPA transformation, platform licensing accounts for 20%–25% of the total costs, while the remaining majority is service-driven. This includes configuration, ongoing management, and maintenance costs, often requiring consultants and RPA specialists for custom development. Furthermore, when business processes or underlying technologies change, substantial costs are incurred for upgrades and reconfigurations, adding complexity and expense to maintain the RPA system’s relevance and efficiency.
In response, some organizations are exploring synchronous AI agent architectures, which promise long-term cost advantages and adaptability to evolving business needs. For now, companies focus on rule-based and diagnostic use cases, keeping AI-driven decision-making and resolutions for future iterations once trust in these synchronous AI agents grows. The big question, though, is whether the ROI justifies the current investment in AI agents. Salesforce charges USD 2 per conversation with its AI agent, while Sarvam plans to bill at 1 cent per minute for its voice bots that can handle multilingual customer service tasks. While these costs might seem steep now, they are expected to decline as AI agents become commoditized, much like what we saw with LLMs. For instance, OpenAI recently reduced the cost of its GPT-4o model to USD 4 per million tokens—a significant drop from the original price of USD 36 per million tokens at its launch in March 2023. This 17-month price reduction marks an average annual decrease of nearly 79%, making advanced AI much more financially accessible. For synchronous AI agents to become widely adopted, similar cost-cutting will be crucial, making the technology more accessible and integrated into everyday business operations.
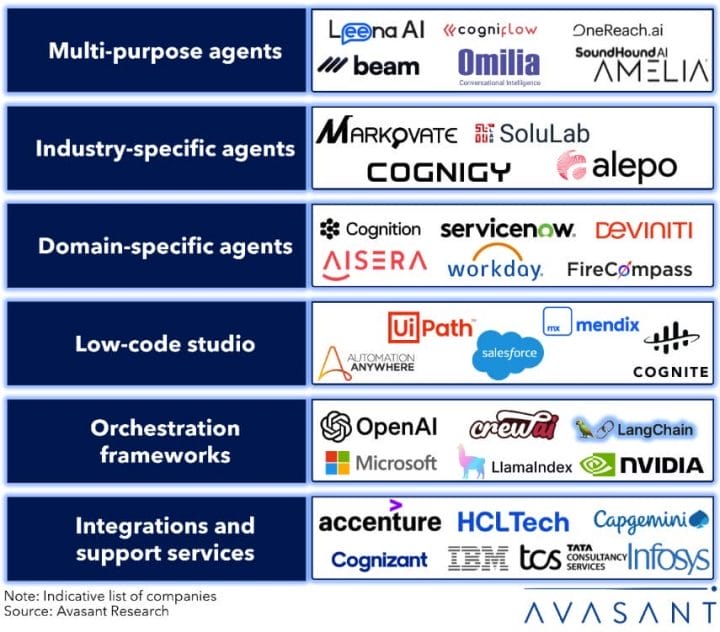
Figure 3: Synchronous AI agent ecosystem
Currently, enterprises have multiple pathways to developing synchronous AI agents, ranging from leveraging prebuilt, domain-specific agents for tasks like order processing or product listing to creating custom AI agents using low-code development platforms or open-source frameworks. The decision hinges on factors such as AI skill availability, data privacy, the level of required customization, and, importantly, the cost.
Way Forward
Looking at the trajectory of automation technologies, it is likely that synchronous AI agents will follow a similar evolution as RPA did years ago. In the early days of RPA, vendors like Automation Anywhere, UiPath, and Blue Prism offered “bot stores,” which allowed companies to buy and deploy prebuilt bots for specific tasks. Over time, as enterprises became more sophisticated in their automation strategies, these vendors helped businesses build their internal bot stores. These custom repositories not only provided faster time to market and reduced licensing costs but also enhanced data privacy and reusability of the automation scripts.
A similar approach seems inevitable for synchronous AI agent architectures. To truly succeed, enterprises will likely begin with proofs of concept (POCs), deploying AI agents that specialize in specific domains such as content creation, data summarization, or acting as research assistants in healthcare. Over time, as these agents demonstrate value, companies will move toward creating internal AI agent repositories. This internal AI agent marketplace would allow enterprises to manage proprietary data securely, ensure access controls safeguarded by internal governance frameworks, and increase convenience by making pre-vetted, trusted AI agents readily available for reuse.
As enterprises embrace this tech and integrate it into their operations, several key considerations emerge when deciding between traditional automation and synchronous AI agents for specific use cases.
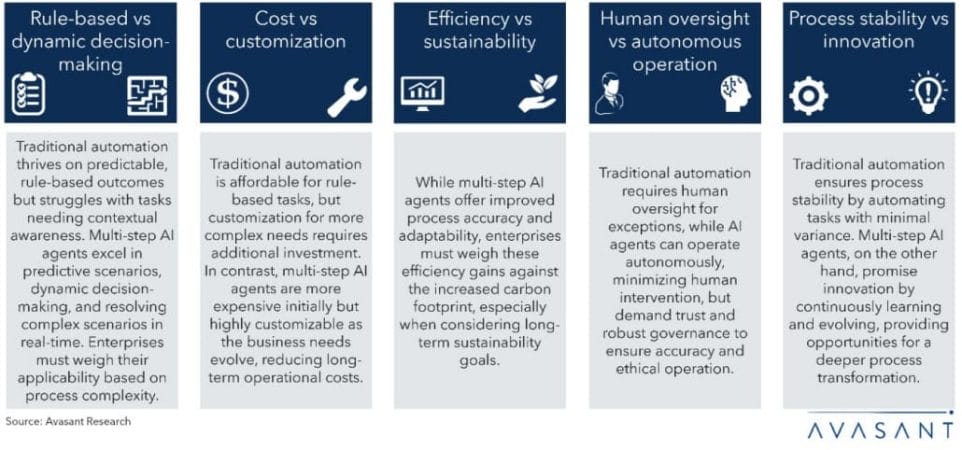
Figure 4: Enterprise considerations for deploying synchronous AI agents
The decision often boils down to whether an organization values immediate efficiency and scalability or prioritizes long-term flexibility and higher ROI. Traditional automation may offer quick wins with low complexity and predictable outcomes, but synchronous AI agents bring advanced capabilities, such as dynamic decision-making and adaptability, that are better suited for evolving and complex business environments. Selecting a balanced approach depends on aligning an enterprise’s short-term operational goals with its long-term strategic vision.
By Chandrika Dutt, Associate Research Director